A CAD Utilizing 3D Massive-Training ANNs for Detection of Flat Lesions in CT Colonography in a Large Multicenter Clinical Trial
A CAD Utilizing 3D Massive-Training ANNs for Detection of Flat Lesions in CT Colonography in a Large Multicenter Clinical Trial
We developed a computer-aided diagnostic (CAD) scheme for detection of flat lesions (also called flat polyps or depressed polyps) in CT colonography (CTC) in a large multicenter clinical trial in collaboration with Don C. Rockey, M.D., at the Southwest Medical Center of the University of Texas. Flat lesions in the colon are a major source of false-negative interpretations in CTC. A major challenge in CAD schemes is the detection of flat lesions, because existing CAD schemes are designed for detecting the common bulbous polyp shape. Our purpose was to develop a CAD scheme for detection of flat lesions in CTC. We developed a CAD scheme consisting of colon segmentation based on histogram and morphologic analysis, detection of polyp candidates based on intensity-based and morphologic feature analysis, and linear discriminant analysis for classification of the candidates as polyps or non-polyps. To detect flat lesions, we developed a tolerantEmorphologic analysis method in the polyp detection step for accommodating the analysis to include a flat shape. For reduction of false-positive (FP) detections, we developed 3D massive-training artificial neural networks (MTANNs) designed to differentiate flat lesions from various types of non-polyps. Our independent database consisted of CTC scans of 25 patients obtained from a multicenter clinical trial in which 15 institutions participated nationwide. Each patient was scanned in the supine and prone positions with collimations of 1.0-2.5 mm and reconstruction intervals of 1.0-2.5 mm. All patients underwent reference-standardEoptical colonoscopy. Flat lesions were determined under either heightE(< 3 mm high) or ratioE(height < 1/2 long axis) criteria. Twenty-eight flat lesions were identified. Among them, 11 (39%) were false negatives in CTC. Lesion sizes ranged from 6-18 mm, with an average of 9 mm. Our MTANN CAD scheme detected 68% (19/28) of flat lesions, including six lesions missedEby reporting radiologists in the original clinical trial, with 10 (249/25) FPs per patient. The figure shows examples of flat lesions, which are very small or on a fold (these are major causes of human misses). Some flat lesions are known to be histologically aggressive; therefore, detection of such lesions is critical clinically, but they are difficult to detect because of their uncommon morphology. It should be noted that these two cases were missedEby reporting radiologists in the original trial; thus, detection of these lesions may be considered very difficult.EOur scheme would be useful for detecting flat lesions which are a major source of false negatives, thus potentially improving radiologistsE sensitivity in their detection of polyps in CTC.
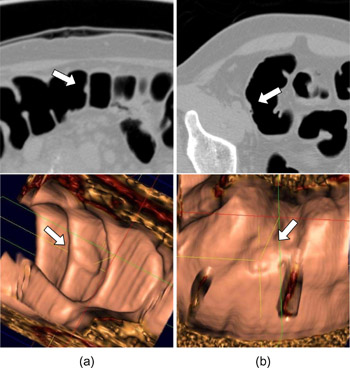
Illustrations of flat lesions which exhibit uncommon flat morphology. (a) A flat lesion on a fold (10 mm; adenoma) in the cecum was detected correctly by our MTANN CAD scheme (indicated by an arrow). (b) A small flat lesion (6 mm; adenoma) in the cecum was detected correctly by our MTANN CAD scheme.