Reduction of Quantum Noise and Radiation Dose in Coronary Angiography by Means of a Neural Filter
We developed a supervised nonlinear filter based on an artificial neural network (ANN), called a “neural filter,” for reduction of quantum noise in coronary angiograms. The neural filter can be trained with input images and the corresponding teaching images. To learn the relationship between low-dose and high-dose x-ray images, we created simulated low-dose angiograms from actual high-dose angiograms by use of a model of quantum noise. We used the simulated low-dose angiograms as the input images and the corresponding high-dose angiograms as the teaching images for the neural filter. After training, the neural filter provided estimated high-dose angiograms. The quantum noise in low-dose angiograms was reduced with the trained neural filter while maintaining the edges of coronary arteries. We compared the performance of the neural filter with that of the leading nonlinear filters. We evaluated the performance of the filters by using the improvement in the signal-to-noise ratio (ISNR). The ISNR was defined as the improvement in the quality of the output images of a filter compared with the input images. An average ISNR of 6.5 dB was obtained with the neural filter for independent test images, whereas an average ISNR of 2.9 dB was obtained with the leading nonlinear filter. With the neural filter, the radiation dose could potentially be reduced by 82%.
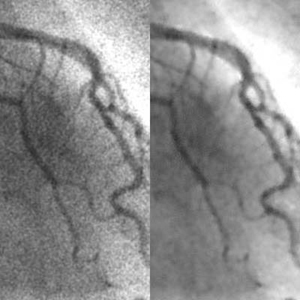
Original low-dose coronary angiogram (left) and the corresponding output image (right) of the trained neural filter for reduction of quantum noise.