Machine/Data Learning
A CAD Utilizing 3D Massive-Training ANNs for Detection of Flat Lesions in CT Colonography in a Large Multicenter Clinical Trial
We developed a computer-aided diagnostic (CAD) scheme for detection of flat lesions (also called flat polyps or depressed polyps) in CT colonography (CTC) in a large multicenter clinical trial in collaboration with Don C. Rockey, M.D., at the Southwest Medical Center of the University of Texas. Flat lesions in the colon are a major source of false-negative interpretations in CTC. A major challenge in CAD schemes is the detection of flat lesions, because existing CAD schemes are designed for detecting the common bulbous polyp shape. Our purpose was to develop a CAD scheme for detection of flat lesions in CTC. We developed a CAD scheme consisting of colon segmentation based on histogram and morphologic analysis, detection of polyp candidates based on intensity-based and morphologic feature analysis, and linear discriminant analysis for classification of the candidates as polyps or non-polyps. To detect flat lesions, we developed a tolerantEmorphologic analysis method in the polyp detection step for accommodating the analysis to include a flat shape. For reduction of false-positive (FP) detections, we developed 3D massive-training artificial neural networks (MTANNs) designed to differentiate flat lesions from various types of non-polyps. Our independent database consisted of CTC scans of 25 patients obtained from a multicenter clinical trial in which 15 institutions participated nationwide. Each patient was scanned in the supine and prone positions with collimations of 1.0-2.5 mm and reconstruction intervals of 1.0-2.5 mm. All patients underwent reference-standardEoptical colonoscopy. Flat lesions were determined under either heightE(< 3 mm high) or ratioE(height < 1/2 long axis) criteria. Twenty-eight flat lesions were identified. Among them, 11 (39%) were false negatives in CTC. Lesion sizes ranged from 6-18 mm, with an average of 9 mm. Our MTANN CAD scheme detected 68% (19/28) of flat lesions, including six lesions missedEby reporting radiologists in the original clinical trial, with 10 (249/25) FPs per patient. The figure shows examples of flat lesions, which are very small or on a fold (these are major causes of human misses). Some flat lesions are known to be histologically aggressive; therefore, detection of such lesions is critical clinically, but they are difficult to detect because of their uncommon morphology. It should be noted that these two cases were missedEby reporting radiologists in the original trial; thus, detection of these lesions may be considered very difficult.EOur scheme would be useful for detecting flat lesions which are a major source of false negatives, thus potentially improving radiologistsE sensitivity in their detection of polyps in CTC.
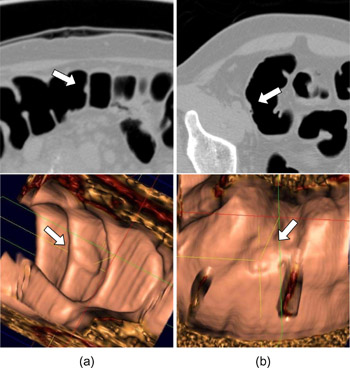
Illustrations of flat lesions which exhibit uncommon flat morphology. (a) A flat lesion on a fold (10 mm; adenoma) in the cecum was detected correctly by our MTANN CAD scheme (indicated by an arrow). (b) A small flat lesion (6 mm; adenoma) in the cecum was detected correctly by our MTANN CAD scheme.
Polyp Detection in CT Colonography: Performance of a CAD Scheme Incorporating 3D MTANNs on False-Negative Polyps in a Multicenter Clinical Trial
We developed computer-aided diagnostic (CAD) scheme for detection of polyps in CT colonography (CTC) and evaluating our CAD scheme with false-negative polyps in a large multicenter clinical trial in collaboration with Don C. Rockey, M.D., the Southwest Medical Center at the University of Texas. A major challenge in CAD schemes for detection of polyps in CTC is the detection of difficultEpolyps which radiologists are likely to miss. Our purpose was to develop a CAD scheme incorporating 3D massive-training artificial neural networks (3D MTANNs) and to evaluate its performance on false-negative (missedE cases in a large multicenter clinical trial. We developed an initial CAD scheme consisting of colon segmentation based on mathematical morphology, detection of polyp candidates based on intensity-based and morphologic feature analysis, and linear discriminant analysis for classification. For reduction of false-positive (FP) detections, we developed a mixtureEof seven expert 3D MTANNs designed to differentiate between polyps and seven types of non-polyps, including folds, stool, the ileocecal valve, and rectal tubes. Our independent database consisted of CTC scans of 614 patients obtained from a large multicenter clinical trial in which 15 institutions participated nationwide. Each patient was scanned in the supine and prone positions with collimations of 1.0-2.5 mm and reconstruction intervals of 1.0-2.5 mm. All patients underwent reference-standardEoptical colonoscopy. One hundred fifty-five patients had clinically significant polyps. Among them, about 45% patients received false-negative interpretations in CTC. For testing our CAD scheme with 3D MTANNs, 14 cases with 14 polyps/masses were randomly selected from the false-negative cases where lesions were visible in both supine and prone scans retrospectively. Lesion sizes ranged from 6-35 mm, with an average of 10 mm. The initial CAD scheme detected 71.4% (12/14) of missedEpolyps, including sessile polyps and polyps on folds, with 18.9 (264/14) FPs per patient. The 3D MTANNs removed 75% (197/264) of the FPs without loss of any true positives; thus, the performance of our CAD scheme was improved to 4.8 (67/14) FPs per patient. With our CAD scheme incorporating 3D MTANNs, 71.4% of polyps missedEby radiologists in the trial were detected correctly, with a reasonable number of FPs. Our CAD scheme would be useful for detecting difficultEpolyps which radiologists are likely to miss, thus potentially improving radiologistsEsensitivity in their detection of polyps in CTC.
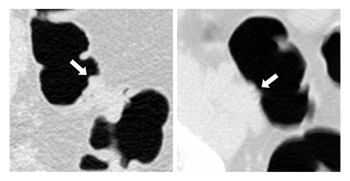
Illustrations of polyps missedEby physicians in a multicenter clinical trial. Left: A very small polyp (6 mm) was detected correctly by our CAD system (indicated by an arrow). This polyp was not detected in either CTC or the gold standardEoptical colonoscopy in the trial. Right: A sessile-type polyp (a major source of human false negatives) was detected correctly by our CAD system.
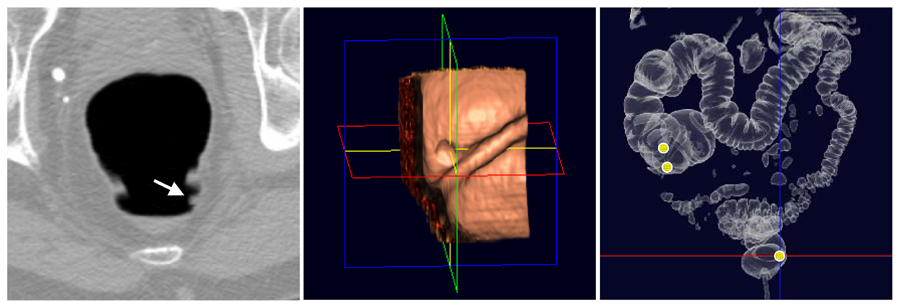
A patient with a small (7 mm) sessile polyp (one of the major sources of false-negative interpretations by radiologists) that was missedEin a clinical trial. (a) our CAD scheme incorporating 3D MTANNs correctly detected the polyp and pointed it by an arrow in an axial CTC image, (b) the polyp in the 3D endoluminal view, and (c) 3D volume rendering of the colon with three computer outputs indicated by yellow circles (one in the rectum is a true-positive detection and the other two are FP detections).