Computerized Detection of Lung Nodules in Low-Dose CT, Part I: Basic Principle of Massive-Training Artificial Neural Network (MTANN) for Reduction of False Positives
We investigated a novel pattern-recognition technique based on an artificial neural network (ANN), called a massive-training artificial neural network (MTANN), for reduction of false positives (FPs) in computerized detection of lung nodules in low-dose CT. The MTANN consists of a linear-output multilayer ANN model, which is capable of operating on image data directly. The MTANN is trained by use of input images together with the teaching images containing the distribution for the likelihood of being a nodule.Enbsp; To achieve a high performance, the MTANN is trained by use of a large number of sub-regions extracted from an input image. The output image is obtained by scanning of an input image with the MTANN, i.e., the MTANN acts like the convolution kernel of a filter. The distinction between a nodule and a non-nodule is made by use of a score which is defined from the output image of the trained MTANN. In the output images of the trained MTANN, nodules are represented by bright pixels, whereas vessels are mostly dark. The MTANN was applied to the task of reducing the number of FPs produced by our computerized scheme for lung nodule detection. Our database contains 50 nodules, including 38 missedEcancers from a lung cancer screening program. The MTANN was trained with ten typical nodules and ten typical non-nodules and then applied to the remaining 40 nodules and 1068 non-nodules (FPs) in a validation test. The results indicated that 66% (706/1068) of the FPs were removed without a reduction in the number of true positives, i.e., a sensitivity of 100% (40 out of 40 nodules) with 0.35 FPs per slice. Accordingly, the false-positive rate of our original scheme was improved from 1.02 to 0.35 FPs per slice without a reduction in the overall sensitivity. Thus, the performance of computer-aided diagnostic schemes for lung nodule detection in CT can be improved substantially by use of the MTANN.
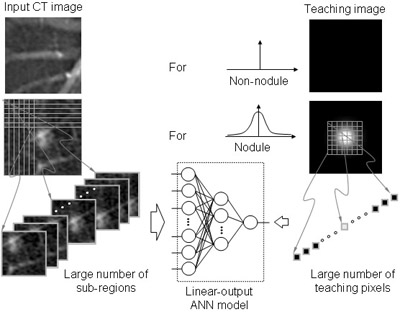
Architecture and training of a massive-training artificial neural network (MTANN) for distinction between nodules and vessels.
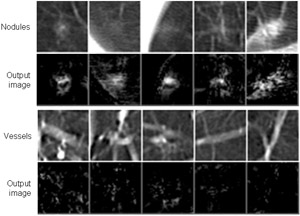
Output images of the trained MTANN for non-training nodules and non-training medium-sized vessels.