Virtual Dual-Energy Radiography: Image-Processing Technique for Suppressing Ribs in Chest Radiographs by Means of Massive-Training Artificial Neural Network (MTANN)
When lung nodules overlap with ribs or clavicles in chest radiographs, it can be difficult for radiologists as well as computer-aided diagnostic (CAD) schemes to detect these nodules. In this study, we developed an image-processing technique for suppressing the contrast of ribs and clavicles in chest radiographs by means of a multi-resolution massive-training artificial neural network (MTANN). An MTANN is a nonlinear filter that can be trained by use of input chest radiographs and the corresponding teachingEimages. We employed boneEimages obtained with a dual-energy subtraction radiography system as the teaching images. For effective suppression of ribs having various spatial frequencies, we developed a multi-resolution MTANN consisting of multi-resolution decomposition/composition techniques and three MTANNs for three different-resolution images. After training with input chest radiographs and the corresponding dual-energy bone images, the multi-resolution MTANN was able to provide “bone-image-like” images which were similar to the teaching dual-energy bone images. By subtracting the bone-image-like images from the corresponding chest radiographs, we were able to produce “soft-tissue-image-like” images where ribs and clavicles were substantially suppressed. The major advantages of our virtual dual-energy radiography compared to “gold standard” duel-energy subtraction radiography are: (1) no additional radiation dose to patients is required, and (2) no specialized equipment for generating dual-energy x-rays is required. Thus, this technique is applicable to any chest radiographs acquired with a standard system for producing soft-tissue images and bone images. Our image-processing technique for rib suppression by means of a multi-resolution MTANN would be useful for radiologists as well as for CAD schemes in the detection of lung nodules on chest radiographs.
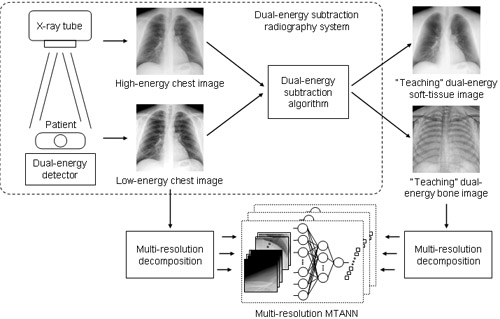
Basic principle of virtual dual-energy radiographyE(VDER) for separating soft tissues from ribs in chest radiographs, consisting of MTANNs and multi-resolution image decomposition/composition. In the training phase, VDER is trained with input chest radiographs and the corresponding teachingEbone images acquired with a commercial dual-energy radiography system (upper figure). VDER can derive soft-tissueEand boneEimages from other standard radiographs based on this training (lower figure).
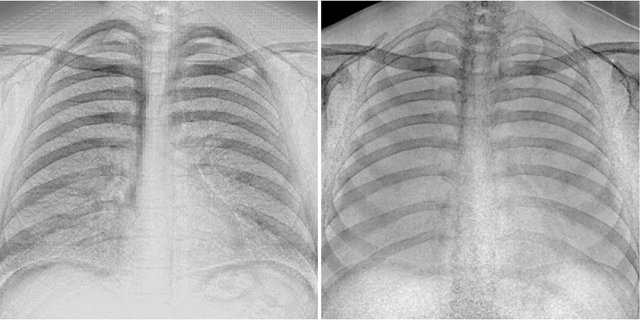
Our virtual dual-energy radiography produces a soft-tissue image (middle image in the upper row) and a bone image (lower left image) from the original chest radiograph (upper left image) which are similar to the corresponding gold-standardE dual-energy radiographs (upper right image and lower right image).