Neural Edge Enhancer for Supervised Edge Enhancement from Noisy Images
We propose a new edge enhancer based on a modified multilayer neural network, which is called a “neural edge enhancer” (NEE), for enhancing the desired edges clearly from noisy images. The NEE is a supervised edge enhancer: through training with a set of input noisy images and teaching edges, the NEE acquires the function of a desired edge enhancer. The input images are synthesized from noiseless images by addition of noise. The teaching edge images are made from the noiseless images by performing the desired edge enhancer. To investigate the performance, we carried out experiments to enhance edges from noisy artificial and natural images. By comparison with conventional edge enhancers, the following was demonstrated: the NEE was robust against noise, was able to enhance continuous edges from noisy images, and was superior to the conventional edge enhancers in terms of the similarity to the desired edges. Furthermore, we have proposed a method for edge localization for the NEE. We compared the NEE, together with the proposed edge localization method, with a leading edge detector. The NEE was proved to be useful for enhancing edges from noisy images.
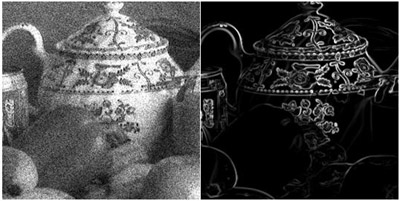
Noisy input image (left) and an ideal edge image (right).
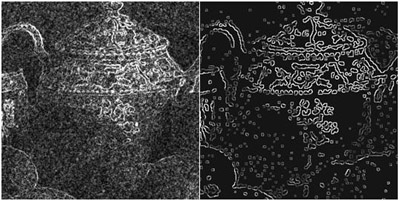
Sobel edge enhancer (left) and Marr-Hildreth edge enhancer (right).
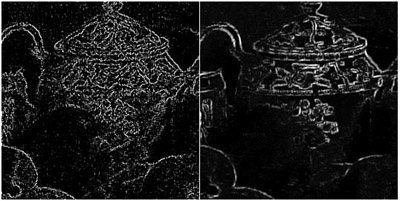
Huckel edge enhancer (left) and neural edge enhancer (right).